How to be more data driven – 4 steps to get you started
It’s well known that being data driven can give your company an advantage. You need to incorporate data thinking into everything you do. But what does that really mean? How do you increase the data maturity of your company? In this short text, I will walk you through four simple steps to help you get you started in data thinking.
Think of a process in your company’s operations that generates data. The following list of questions will help you figure out what data your activities generate and how usable that data is. Your answers will also give you an idea of your level of data maturity, or “data drivenness” as I like to call it.
The four steps to get you started in data thinking:
- Process. Is your process a true (“native”) digital process, or an analogue process using digital tools?
- Data type. What kind of data does this process generate?
- Data format. Is the generated data in a format that can be directly used for analytics and combined with other data in an automated manner?
- Data storage. Is the generated data stored in a way that it is easily transferable into a common data storage?
Being data driven means that you systematically use analysed data to drive your business. Analysing is just another word for “combine and compare”. In other words, your data needs to be in a format that can be combined and compared with other data you’ve gathered.
For example, your business goals may require you to compare data that seems incomparable at first glance, i.e., to compare “apples and oranges”. Contrary to the popular saying, you can indeed compare “apples and oranges”. However, to be able to compare them, you need to figure out what they have in common, like that they’re both fruits. Once you’ve categorized them as fruit, you can compare them as different kinds of fruit. Same goes for, say, contacts: some are ‘customers’ while some are ‘suppliers’. You probably also have different kinds of customers.
What is a native digital process?
To answer the first question in the list, have a look at your processes. If you use modern digital tools, such as online SaaS tools, your processes may appear digital. However, a process using digital tools may still be analogue. Here’s an example: You have an online sales tool, where your sales reps log the deals they make. Now surely this is a digital process, as the sales reps use the tool through a mobile app, on the go? Well, it depends.
If the process were the same even if the sales reps wrote the deal info in a paper notebook, then the process is an analogue process. Just using a digital tool instead of an analogue tool does not make the process a digital process.
There are many ways to rework an analogue sales process into a native digital process. You could have the sales tool “listening” to the conversation between your sales rep and the client, and use natural language processing (NLP) to identify and record the deal information without any manual input by the sales rep. For example, when the customer says, “I’ll buy a hundred oranges, if you can deliver them by Friday”, and the sales rep answers, “Great, you’ll get a 5% discount on that”, the tool will log the following data:
[Product: Oranges; Quantity: 100; Requested delivery data: Friday; Pricing term: -5%]
Note that NLP is a digital tool. The process becomes a native digital process when you design the process so that it maximises the benefits of using the capabilities of the digital tools.
Do you generate usable data?
This bring us to questions 2, the kind of data generated, and question 3, is the data in a usable format. You’ve might have heard of the saying “data is the new oil” and just like crude oil, raw data is for the most parts unusable with out refining.
One common problem is a mismatch of units. For instance, there are three different types on ‘tons’ (the US ton, the Imperial ton, and the metric ton) and if your sales data includes information in plain text form that reads “a ton of apples delivered in a few days” it’s ambiguous as best to interpret how many pounds or kilograms of apples the statement refers to. It is also difficult to convert “a few days” to delivery dates.
So, you see, just generating data is not enough. You need to plan for what kind of data you get out, and check that it’s usable. If the same sales data “a ton of apples delivered in a few days” is coupled with a table for units that determine the type of ‘ton’ and has a range for ‘a few days’ the situation change dramatically and makes the data usable for both operations and analytics.
Why is a common data storage important?
Finally, we arrive at question 4. Your data needs to be stored in a way that it’s easily retrievable by the analytics pipeline. In many cases where the data comes from several sources, you need to combine it to be able to extract significant insights from analysis.
Companies frequently use vast sets of different online tools combined with some (on-premise) legacy systems. By doing so, you may end up in a situation where you have a lot of data scattered in multiple repositories. In the worst case, the combination of the scattered data is done by manually copy-pasting the data into a spreadsheet.
Manual data gathering and combining is time consuming, error prone and person dependent. With proper data architecture, all your data flows into a common data repository, like a data lake, where it is engineered to be in a combinable and comparable format, ready to be utilized by automated or ad hoc analytics.
Have another look at the four steps I listed above. How would you answer the questions? Is there something you would add to the list?
If you would like to know more about ways to enhance your data drivenness, drop us a line.
About the author
Roger Sittnikow is a Senior Advisor on data projects and Executive MBA with a passion for financial and business analytics, with over 30 years of leadership experience.
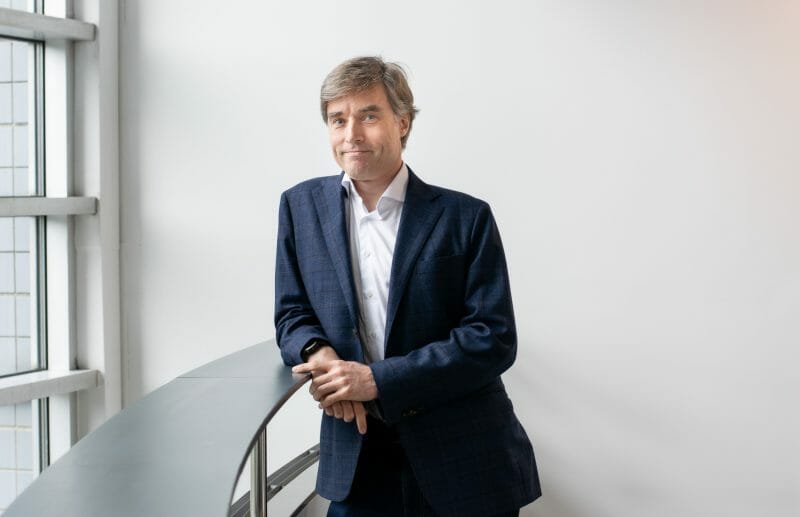